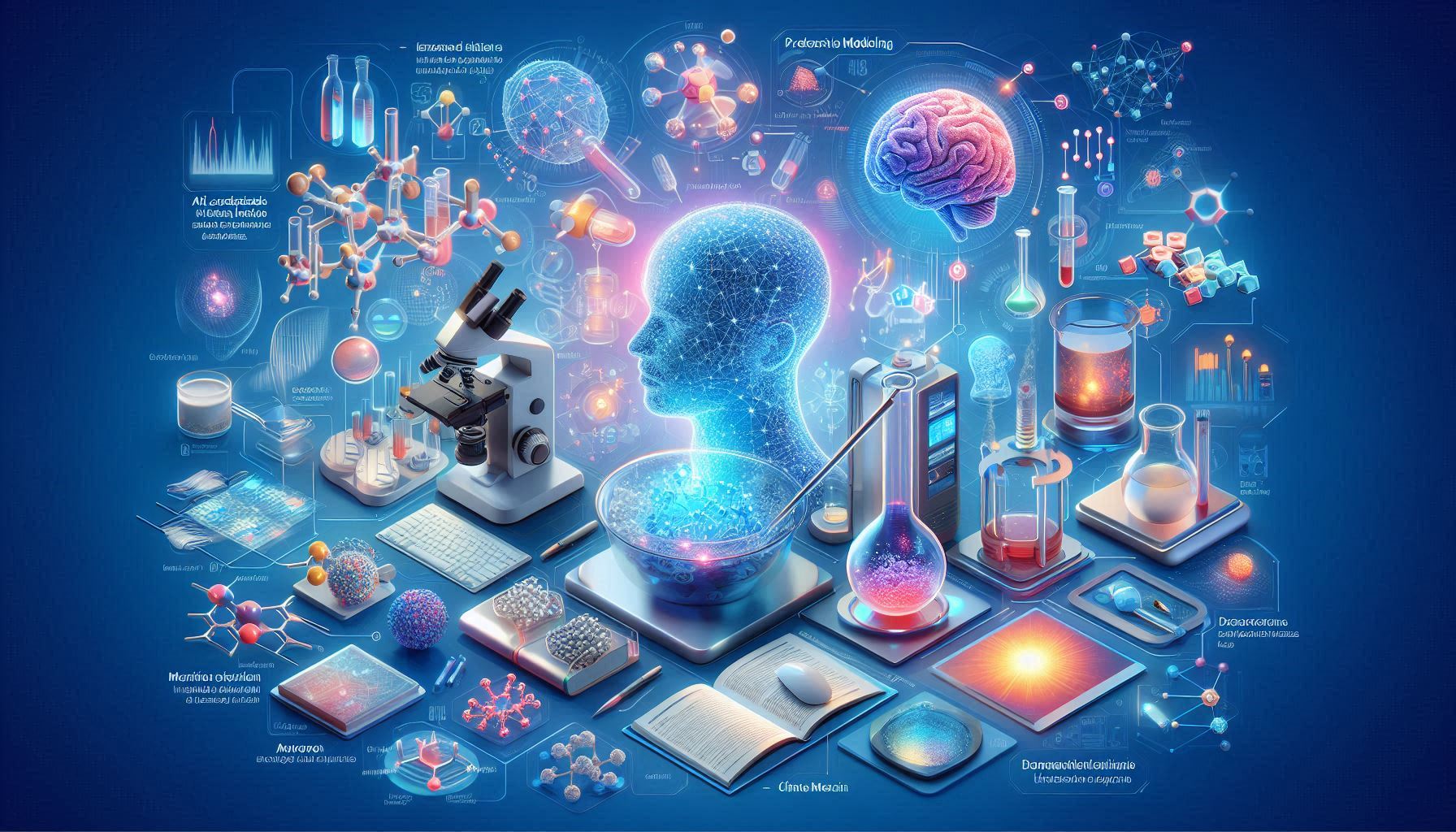
Revolutionizing Discovery: The Future of Scientific Research with Artificial Intelligence
The incorporation of artificial intelligence (AI) into scientific research has heralded a transformative era of discovery, characterized by unparalleled advancements in data analysis, predictive modeling, and interdisciplinary collaboration. In the past decade, AI has shifted from being a speculative concept to a tangible tool, accelerating innovation across various domains, from healthcare to climate science. Recent breakthroughs, encompassing AI-driven materials discovery, genomic modeling, and climate prediction systems, illustrate the technology's potential to address some of humanity's most pressing challenges. Looking ahead over the next five years, AI is set to further revolutionize scientific inquiry by enhancing the precision of simulations, enabling personalized medical interventions, and fostering collaborations that cut across traditional disciplinary borders. This report delves into these developments, considering both the current state of AI in research and its transformative potential by 2030.
Accelerating Materials Discovery Through Generative AI
Foundations of AI-Driven Materials Science
The search for novel materials—crucial for advancements in energy, healthcare, and sustainability—has long been hampered by the slow, trial-and-error approach of experimental research. Generative AI models are breaking these barriers by mastering the "language" of molecular structures and predicting new compounds with desired properties. Collaborations, such as those between Microsoft Research and academic and industrial partners, have developed foundation models trained on extensive datasets of known materials. These models quickly generate candidate materials for superconductors, carbon capture substances, and pharmaceutical compounds. By using transformer architectures similar to those in natural language processing, these models treat atomic configurations as sequences to decode. Simulating quantum mechanical interactions at scale, AI technology reduces dependency on costly lab experiments, significantly shortening discovery timelines from years to months.
Case Study: Energy Storage Innovations
One promising application is in the realm of next-generation batteries. Generative models have identified novel solid-state electrolytes with ionic conductivities surpassing traditional lithium-ion solutions, resolving crucial challenges in energy density and safety. For example, a 2024 study utilized AI to screen over 100,000 potential compositions, narrowing them down to 12 high-probability candidates, which were then validated through synthesis. By 2030, such approaches are predicted to dominate materials research, with AI not only suggesting new structures but also optimizing synthesis pathways and predicting environmental impacts. This shift could enable the commercialization of fusion reactor materials, room-temperature superconductors, and biodegradable polymers—advancements previously considered to be decades away.
Revolutionizing Healthcare Through Multimodal AI Systems
Enhanced Diagnostics and Radiology
In medical imaging, AI is radically changing the way clinicians interpret complex datasets. An example of this technological leap is the RAD-DINO model, stemming from a partnership between Mayo Clinic and Microsoft Research. By integrating text and image data from chest X-rays, the system automates report generation, detects misplaced medical devices, and identifies subtle changes across sequential scans. Early trials revealed a 40% reduction in diagnostic errors for pneumonia detection and a 30% improvement in tumor progression tracking. Over the next five years, such models will expand to include real-time sensor data from wearable devices, enabling dynamic risk assessments for conditions like heart disease and diabetes.
Predictive Analytics in Autoimmune Diseases
AI’s ability to analyze longitudinal health data is transforming the management of chronic diseases. A 2025 study by Penn State researchers demonstrated an AI model capable of predicting rheumatoid arthritis progression five years before symptoms appear, with 92% accuracy—surpassing conventional biomarkers by a wide margin. The system synthesizes electronic health records, genetic predispositions, and environmental factors to categorize patients into risk groups, guiding preventive therapies. By 2030, similar frameworks will likely extend to diseases like lupus, multiple sclerosis, and type 1 diabetes, shifting the healthcare paradigm from reactive treatment to preventive strategies.
Climate Modeling and Sustainability Solutions
High-Resolution Climate Simulations
Existing climate models often fall short in resolving fine-scale processes like cloud formation and ocean eddies, introducing uncertainties into long-term projections. AI addresses these gaps through hybrid models that merge physics-based equations with machine learning surrogates. Projects like NVIDIA’s Earth-2 initiative employ generative adversarial networks (GANs) to simulate atmospheric dynamics at a kilometer scale—a 1000-fold improvement over traditional models. These tools have enabled the accurate prediction of events such as the unprecedented Mediterranean heatwave of 2024, six months in advance, highlighting their potential for disaster preparedness. In the coming years, federated learning systems will amass data from global sensor networks, creating real-time, interactive climate dashboards for policy makers.
Optimizing Renewable Energy Systems
AI-driven optimization is speeding up the transition to sustainable energy infrastructures. In wind farm design, reinforcement learning algorithms now adjust turbine placements according to real-time weather data, enhancing energy output by 15–20%. Solar grid management systems utilize computer vision to detect panel degradation and prioritize maintenance, minimizing downtime by 30%. Looking forward, integrating AI with quantum computing could solve daunting problems related to nuclear fusion plasma containment, possibly unlocking limitless clean energy by the 2030s.
Decoding Biological Complexity Through Genomic AI
The Evo 2 Model and Generative Biology
The Evo 2 AI framework signifies a dramatic advance in genomic analysis. Trained on a staggering 9.3 trillion nucleotides from 128,000 species, this model predicts the functional impact of genetic mutations and designs synthetic genomes with bacterial complexity. A landmark experiment in 2025 saw Evo 2 engineer a novel bacteriophage capable of targeting antibiotic-resistant Staphylococcus aureus, achieving 99% efficacy in vitro. These capabilities suggest a future where AI-driven “generative biology” routinely produces customized microbes for applications in carbon sequestration, bioremediation, and personalized probiotics.
CRISPR-AI Synergy in Gene Editing
Precision gene editing is undergoing a revolution via AI systems that predict off-target effects and optimize guide RNA sequences. DeepMind’s AlphaFold 3, when integrated with CRISPR-Cas9 databases, now attains single-nucleotide resolution in editing planning, reducing unintended mutations by 80% compared to human-led methods. By 2030, these tools may enable the correction of complex polygenic disorders like schizophrenia and Alzheimer’s, surpassing today’s focus on monogenic conditions.
Fostering Interdisciplinary Collaboration via AI Platforms
Expert Matching and Knowledge Synthesis
AI platforms such as Scinapse’s Expert Finder are dismantling traditional academic silos by connecting researchers across disciplines. By analyzing an immense corpus of 50 million publications and patent filings, these systems identify complementary skill sets. A trial at Stanford in 2024 demonstrated that AI-matched teams produced 65% more high-impact papers than self-assembled groups, with notable gains in areas such as bioinformatics and nanotechnology.
Virtual Research Environments
Immersive simulation technologies, expected to grow rapidly through 2030, are creating virtual laboratories where geographically dispersed teams can collaborate in real time. Surgeons in Nairobi can now practice rare procedures in VR environments modeled on operating rooms from Boston hospitals, while climate scientists can visualize 4D atmospheric models via AR headsets. These platforms, enhanced by AI tutors suggesting experimental parameters, democratize access to cutting-edge research infrastructure.
Ethical Considerations and Future Challenges
Bias Mitigation in AI-Driven Research
As AI becomes more integral to hypothesis generation, concerns about algorithmic bias and reproducibility have emerged. A 2025 analysis of AI-predicted drug candidates revealed a 40% overrepresentation of molecules soluble in dimethyl sulfoxide (DMSO)—a solvent preferred in historical datasets but unsuitable for clinical use. Addressing such issues necessitates transparent model architectures and adversarial validation techniques that stress-test predictions against diverse datasets.
Regulatory Frameworks for AI-Generated Discoveries
Current intellectual property laws are ill-equipped to handle inventions jointly created by humans and AI. The 2024 “Evo Bacteriophage” case exemplifies emerging legal ambiguities regarding attribution between AI systems and their developers. By 2030, an international consensus on liability frameworks, data sovereignty, and ethical guidelines for autonomous research systems will likely form—a critical step for sustaining public trust in AI-enhanced science.
Conclusion
The integration of artificial intelligence into scientific research represents a paradigm shift akin to the invention of the microscope or the computer. Over the next five years, AI will evolve from being a specialized tool to becoming a ubiquitous collaborator, enhancing each phase of the scientific method—from hypothesis generation to peer review. Breakthroughs in personalized medicine, climate resilience, and materials science will accelerate as multimodal AI systems integrate insights from previously disconnected disciplines. However, realizing this potential demands proactive measures to ensure equitable access, algorithmic transparency, and ethical oversight. As researchers increasingly partner with AI, the scientific enterprise is poised to enter its most productive and impactful era yet.